Understanding Image Annotation: A Comprehensive Guide
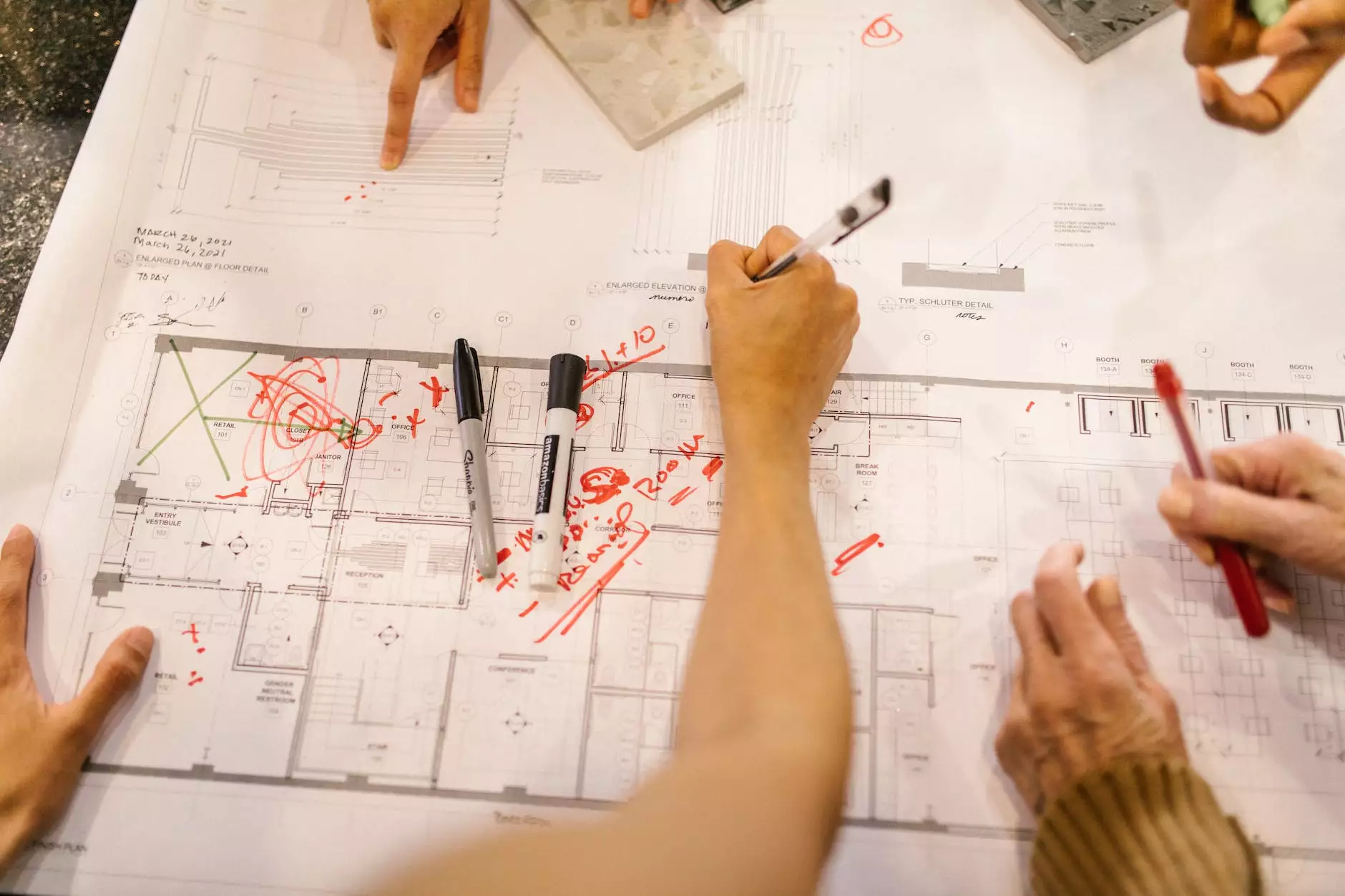
Image annotation plays a crucial role in the development of robust machine learning models and artificial intelligence systems. As businesses increasingly turn to data-driven methodologies, understanding the intricacies of image annotation can significantly enhance the quality and accuracy of their AI initiatives. In this article, we will explore the various facets of image annotation, delve into the essential tools available in the market, and examine how Keylabs.ai stands at the forefront of this critical process.
The Importance of Image Annotation in AI
In the realm of artificial intelligence, particularly in computer vision, image annotation is the foundation upon which models are built. It involves labeling images for the purpose of training AI algorithms to recognize patterns, classify data, and make predictions. Here are some key aspects of the importance of image annotation:
- Training Data Preparation: Accurate annotations are crucial for creating a reliable training dataset. Without precise labels, the machine learning model may fail to learn effectively.
- Enhanced Model Accuracy: Properly annotated images can lead to drastically improved accuracy in AI systems, resulting in better performance on real-world tasks.
- Facilitating Understanding: Image annotations help in breaking down complex visual information, making it easier for AI models to comprehend and act upon it.
- Industry-Specific Applications: Many sectors, including healthcare, automotive, and security, rely heavily on image annotation for tasks ranging from tumor detection to autonomous driving.
Types of Image Annotation Techniques
Understanding the various techniques of image annotation is essential for selecting the right method for your project. Here are some commonly used techniques:
1. Bounding Box Annotation
This technique involves drawing a rectangle around the object of interest within an image. It is widely used in object detection applications where pinpoint location is vital.
2. Polygon Annotation
Polygon annotation allows for more precise outlines of objects by using multiple points to create a shape. This is particularly useful in scenarios where objects are irregularly shaped.
3. Semantic Segmentation
In semantic segmentation, each pixel in an image is labeled with a class. This technique is frequently applied in image segmentation tasks where it's essential to understand the context of every pixel.
4. Landmark Annotation
Used mainly for facial recognition and biometrics, this method places points on specific features of a given image, such as eyes, nose, and mouth.
Data Annotation Tools and Platforms
As the demand for quality image annotation grows, various tools have emerged to facilitate the process. Below are some of the leading data annotation tools and platforms:
Keylabs.ai
Keylabs.ai offers a state-of-the-art data annotation platform that excels in providing high-quality image annotations. Its user-friendly interface, intuitive tools, and collaborative features make it ideal for businesses looking to streamline their data preparation processes.
Labelbox
Labelbox is another powerful platform that allows users to create, manage, and optimize training datasets at scale. With a focus on annotation efficiency, Labelbox integrates machine learning processes to automate repetitive tasks.
Amazon SageMaker Ground Truth
A part of the Amazon Web Services suite, SageMaker Ground Truth offers a fully managed data labeling service, enabling users to build highly accurate training datasets quickly and affordably.
CVAT
Computer Vision Annotation Tool (CVAT) is an open-source tool developed by Intel that allows users to annotate images and videos effectively. CVAT is highly customizable, making it suitable for various data annotation scenarios.
Choosing the Right Image Annotation Tool
When selecting a data annotation tool, consider the following factors:
- Ease of Use: Ensure the tool has a user-friendly interface that minimizes the learning curve for your team.
- Collaboration Features: Choose a platform that facilitates teamwork, allowing multiple users to work on annotations simultaneously.
- Scalability: As your projects grow, the tool should be able to handle increased workloads without compromising performance.
- Integration Capabilities: Look for tools that easily integrate with your existing systems and data workflows.
Best Practices for Image Annotation
To achieve high-quality image annotation, it’s essential to adhere to best practices that enhance both efficiency and accuracy:
1. Define Clear Guidelines
Establishing clear annotation guidelines ensures consistency across your team. A well-defined process helps maintain high-quality standards throughout the project.
2. Utilize Training Sessions
Before starting annotation, conduct training sessions to familiarize the team with tools and techniques specific to your project requirements.
3. Implement Quality Control Measures
Integrating quality control checkpoints in the annotation process can significantly reduce errors. Implementing peer reviews and validation checks enhances accuracy.
4. Keep Your Annotations Consistent
Uniformity is key in image annotation. Ensure that all annotators are following the same conventions to avoid discrepancies that can confuse the model.
The Future of Image Annotation
As technology continues to evolve, the future of image annotation looks promising. Emerging trends such as automated annotation powered by artificial intelligence and the growing adoption of deep learning models are reshaping the landscape. Key innovations include:
- AI-Powered Annotations: Machine learning models can assist or even automate parts of the annotation process, drastically reducing the time and effort involved.
- Real-Time Annotation: Innovative tools are being developed that facilitate real-time annotations, enhancing collaboration and efficiency.
- Enhanced User Interfaces: Future platforms are likely to provide even more intuitive interfaces, enabling annotators to work more effectively.
- Integration with IoT Devices: As IoT devices proliferate, the need for real-time image and data annotation in applications such as surveillance and healthcare will continue to grow.
Conclusion
In conclusion, image annotation is not just a technical process; it is a critical component that dictates the success of machine learning and AI initiatives. Understanding the various techniques, tools, and best practices ensures that businesses can leverage data effectively to drive innovation and efficiency. Keylabs.ai exemplifies a platform that empowers organizations to harness the full potential of data annotation, offering customizable solutions tailored to a myriad of needs. As we move into a future increasingly defined by AI, the importance of high-quality image annotation will only continue to rise.
Your organization’s journey in the AI landscape starts with understanding and implementing effective image annotation. Invest in the right tools and practices today to lead your business towards tomorrow’s technological advancements.