Enhancing Business Efficiency with Labeling Tool Machine Learning
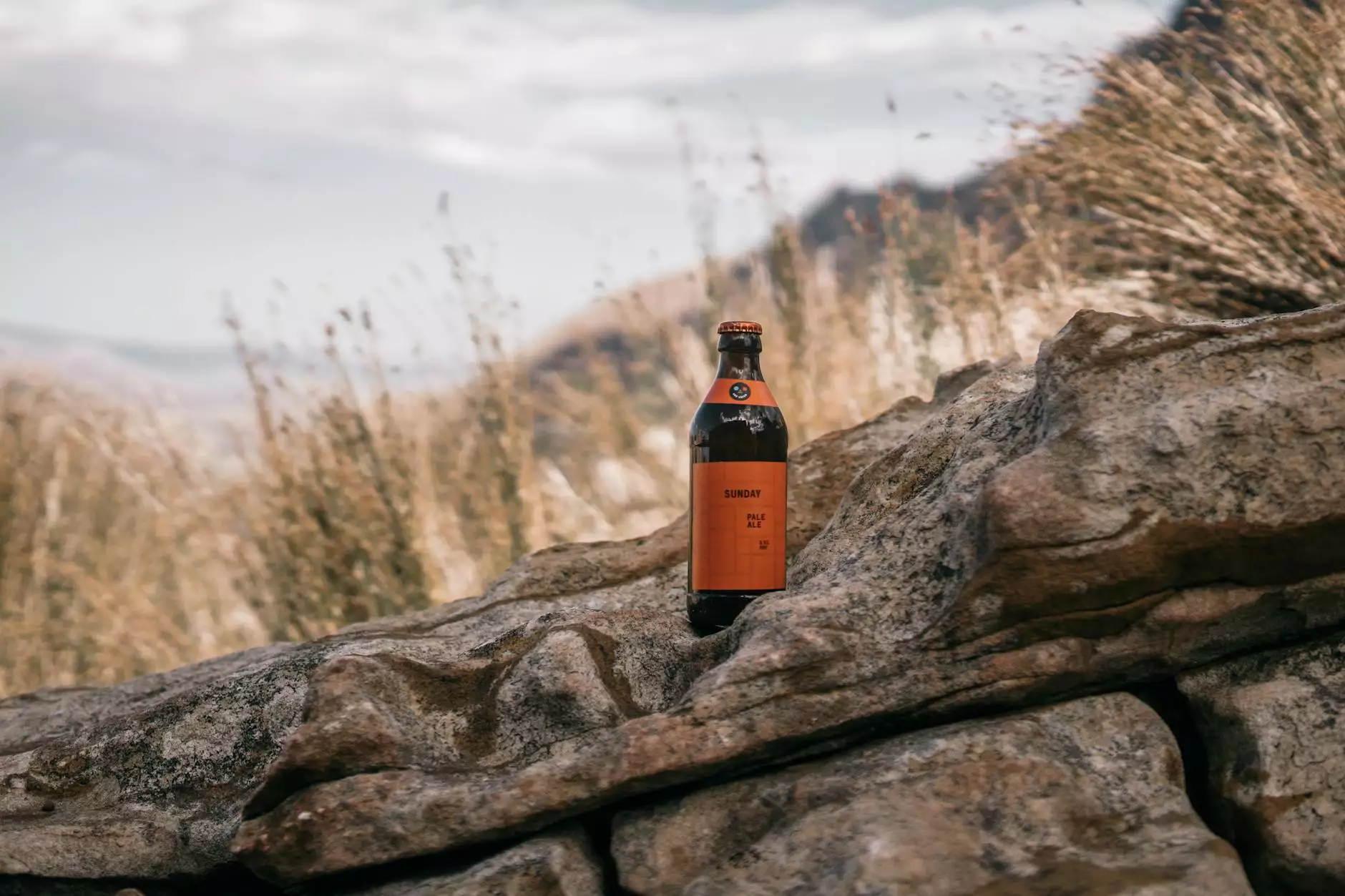
In today's digital world, the ability to accurately and efficiently process vast amounts of data is paramount for businesses seeking a competitive edge. One crucial aspect of this process is the labeling tool machine learning, which plays a pivotal role in data annotation, enabling companies to make sense of big data and drive actionable insights.
Understanding Data Annotation and Its Importance
Data annotation is the process of labeling data for machine learning models. This labeled data serves as the foundation upon which models learn and make predictions. Businesses across various sectors, from healthcare to finance and e-commerce, rely heavily on data annotation to train their algorithms, ensuring they can accurately interpret information.
The Role of Machine Learning in Data Annotation
Machine learning (ML) has revolutionized the way data is processed and understood. By utilizing algorithms, businesses can automate the labeling process, significantly reducing both time and labor costs. Here, labeling tool machine learning comes into play as a crucial component of this automation.
- Efficiency: Automated tools streamline the annotation process, leading to faster turnaround times.
- Consistency: Machine learning ensures that label quality remains high and consistent across the dataset.
- Scalability: As data grows, machine learning can scale up its operations without a hitch, accommodating expansive datasets.
Benefits of Using Labeling Tool Machine Learning
The implementation of labeling tool machine learning offers several advantages to organizations. Below are some of the most notable benefits:
1. Accelerated Data Processing
With machine learning models capable of processing data at a rapid pace, businesses can achieve quicker insights. This swift processing is particularly beneficial in industries where time-sensitive decisions are critical.
2. Cost-Effectiveness
Investing in automated labeling tools reduces the need for extensive manual labor. This leads to reduced operational costs and allows companies to allocate resources to other important areas.
3. Enhanced Accuracy
Machine learning algorithms improve over time, meaning that as they process more data, they become increasingly accurate. This enhancement is crucial for businesses that depend on precision in their models.
4. Improved Data Quality
Using sophisticated algorithms, businesses can ensure that their data is clean and well-labeled, which leads to better model performance. High-quality labeled data translates to better insights and outcomes.
Implementation of Labeling Tools for Machine Learning
To leverage labeling tool machine learning effectively, businesses should consider the following steps for implementation:
Step 1: Identify the Data Needs
Before deploying a labeling tool, it’s essential that organizations first identify the type of data they need to annotate. Understanding whether the data is text, image, or audio will determine the nature of the labeling tool required.
Step 2: Choose the Right Tool
There are numerous labeling tools available, each offering a distinct set of features. Companies should evaluate tools based on factors such as scalability, supported data types, and integration capabilities with existing systems. Keylabs.ai offers advanced options in this regard, positioning businesses for success.
Step 3: Training the Machine Learning Model
Once the tool is in place, the next step is to train the machine learning model. This involves feeding the labeled data back into the system to refine its learning process. Regular feedback loops can dramatically improve model accuracy.
Step 4: Validate and Test
Validation is critical in machine learning. Organizations should validate their models against a test dataset to ensure that the predictions made are accurate and consistent with expected outcomes.
Case Studies: Success Stories in Labeling Tool Machine Learning
To better understand the power of labeling tool machine learning, let’s look at some real-world applications:
Healthcare Sector: Diagnosis Support Systems
In the healthcare sector, companies have employed machine learning labeling tools to augment diagnostic support systems. By accurately labeling medical images, they can train models that assist healthcare professionals in diagnosing conditions more reliably.
Retail Industry: Customer Behavior Analysis
Retailers have utilized data annotation tools to better understand customer behavior. By labeling transaction data and customer interactions, machine learning models can predict future buying patterns, thus enabling personalized marketing strategies.
The Future of Labeling Tool Machine Learning
The future of labeling tool machine learning is exciting and holds immense potential for businesses. As technology continues to evolve, we can expect:
Increased Automation
Automation will continue to be a significant driver in the data annotation space. As machine learning algorithms become more sophisticated, the need for human intervention in the labeling process will diminish.
Greater Integration with AI
As artificial intelligence (AI) progresses, labeling tools will integrate seamlessly with other AI technologies. This integration will lead to more holistic approaches to data analysis and decision-making.
Conclusion
The integration of labeling tool machine learning into business processes represents a vital step forward in the digital transformation journey. As organizations harness this technology, they can expect not only improved operational efficiency but also enhanced decision-making capabilities based on high-quality data.
As industries continue to evolve, adopting advanced labeling tools will not just be an option; it will soon become a necessity for companies looking to thrive in the data-driven economy.
By utilizing platforms such as Keylabs.ai, businesses can find the tools they need to navigate this landscape effectively and position themselves for future success.